"The 'Electricity' of Modern Times."
What actually is AI and where is it going?
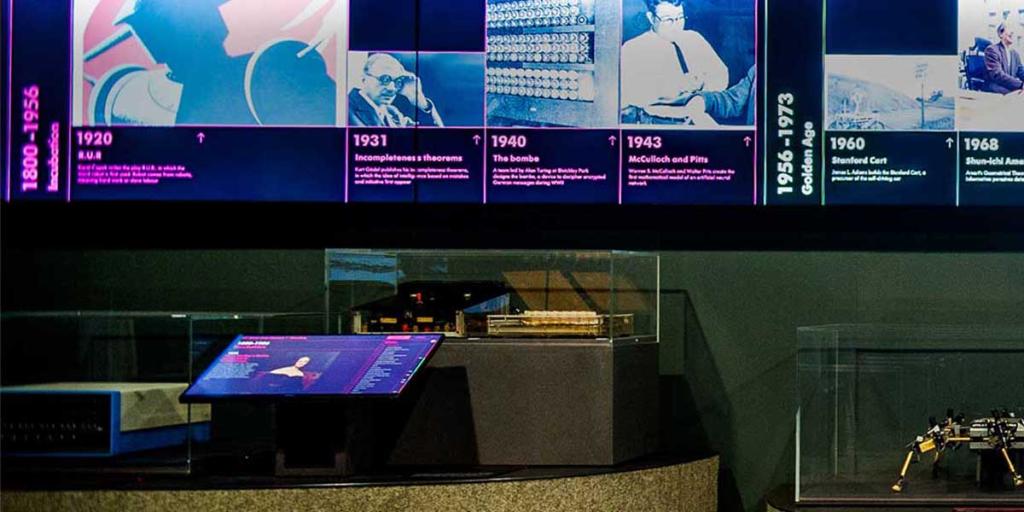
Artificial Intelligence (AI) is about trying to make computers do things that we, as humans, consider intelligent. The term AI was coined in 1956 and the aim has remained essentially unchanged since then. An early focus of AI was to create computer programmes that could play chess well. In the beginning these programmes played poorly. It took 40 years for chess programmes to reach, and then soon after exceed, the quality of grandmasters, with a famous victory of IBM's DeepBlue programme over Gary Kasparov in 1997.
AI is not only about thinking and playing games, but also about getting robots to do things that humans can do. An early example is Shakey the Robot, developed at Stanford in the late 1960s. It could perceive its environment using video cameras, and had bumpers to detect obstacles. It operated in an office building and could be given an aim, like going to a particular office to collect an object. It would then come up with a plan, which it would then execute.
Shakey was very slow and often got stuck. Robots have a come a long way since then and have many uses, from factory production lines, to self-driving cars, to robots that help after disasters by going into areas too dangerous for humans. However, while chess has been 'solved' with super-human chess programmes that never lose to humans, robots are still far behind. This is perhaps not surprising. While chess is a 'single task', to emulate humans, robots have to do many things: perception (understanding their environment), planning and reasoning (how should the robot try and reach a goal), and communicating.
How is AI created?
In the example of chess, the game can be thought of as a planning problem, where the chess AI is created like a normal computer programme. The chess AI uses 'brute force' and considers a huge number of future possible positions, and then chooses a move using rules of thumb. The main reason that it took so long to develop strong chess programmes is due to the sheer number of possible different ways that a game may play out (discover the mind blowing number here). Dealing with all of these cases takes a lot of computing power.
"The way that Machine Learning solves this problem is very similar to how we teach a child to read."
However, perception and communication are very different and it is not possible to write down explicit rules as we do for chess. The approach that has worked for these problems is 'learning by example', in fact learning from tonnes of examples, a sub-field of AI called 'Machine Learning'. The big progress in AI over the last 15 years has been due to improvements in this area.
In the simplest case, Machine Learning works like this. Suppose that we would like to create a programme to help the post office sort letters by recognising postcodes. Handwriting styles and quality can vary dramatically and indeed even humans can sometimes struggle to read other's writing. The way that Machine Learning solves this problem is very similar to how we teach a child to read. We show the machine lots of examples of written postcodes and tell it how each should be read. Over time, the more examples it sees, the better it gets at reading the postcodes correctly.
For the ancient Japanese game of Go, the number of possible moves and board positions is much larger than in Chess. This meant that brute force approaches cannot work and a biologically-inspired Machine Learning approach called 'neural networks' was needed. In 2016, in a series of matches that rocked the world of Go (and AI), a new programme called AlphaGo created by UK AI startup DeepMind beat Lee Sedol, one of the best players in the world. A fascinating story you can watch in 'AlphaGo - The Movie'.
Where are we going with AI?
The examples we have seen so far all relate to quite specific tasks, like playing Go or recognising postcodes - this is often called narrow AI. Most AI researchers focus on solving particular problems like this. Narrow AI is all we have so far, however, the ultimate goal of AI is much grander: creating strong AI, a computer program that can do any task that a human can. While narrow AI systems perform very well when they encounter normal situations, they struggle as soon as something unexpected happens, which actually happens quite often! To deal with this, strong AI will need common sense and the ability to reason by analogy.
As an example, self-driving cars can work perfectly under normal driving conditions, but they struggle when they encounter situations that are too far from those that they were trained on. This resulted in a self-driving Tesla car driving straight into a street-cleaning truck in China. The car had been trained in Europe and had simply never seen a truck that looked like this one before. The self-driving car doesn't really understand what is happening around it, nor have the common sense to realise that the truck should be avoided. This particular problem may be easy to fix by more training, but there is always some new unexpected situation that would confuse a system without genuine common sense.
"The ultimate goal of AI is much grander: creating strong AI, a computer program that can do any task that a human can."
Will we actually be able to create strong AI, and if so when?
AI researchers have been terrible at predicting the timeline of progress. For example, Marvin Minsky, a famous AI researcher, said in 1967, "within a generation... the problem of creating 'Artificial Intelligence' will substantially be solved". Then in 1970, "in from three to eight years we will have a machine with the general intelligence of an average human being." While Minsky was spectacularly wrong, with strong AI probably still a long way away some 50 years later, unexpected breakthroughs are always possible. For example, before DeepMind created AlphaGo in 2016, many AI researchers had publicly stated that we were decades from being able to beat professional Go players.
This is not an isolated example of a breakthrough. The large neural networks used in AlphaGo have also led to a number of other breakthroughs over the past 15 years. Similar to the 1960s and 70s, the 21st century is so far a period with much optimism about current and future AI. In 2007, AI researcher Andrew Ng lauded AI as "the 'new electricity' ... just as electricity transformed many industries roughly one hundred years ago; AI will also now change every major industry."
While there is no doubt that AI is transforming many aspects of our lives, there is a danger that we get caught up in the hype. AI entrepreneur and writer, Gary Marcus, is sure AI leaders and the media are exaggerating the field's progress. Besides, with regards to the ultimate goal of strong AI, AI entrepreneur Erik Larson points out that "success with narrow applications gets us not one step closer to general intelligence."
"Just as electricity transformed many industries roughly one hundred years ago; AI will also now change every major industry."
While this debate rumbles on, what can be certain is that even if AI's current influence is oversold, it will affect all of us in the future and indeed, is already being used in more ways than you might realise.